Internet of Things Access Technologies
In today's interconnected world, the Internet of Things (IoT) has become a significant part of our lives. From smart homes to industrial automation, IoT devices rely on access technologies to establish connectivity. This blog post aims to delve into the world of IoT access technologies, understanding their importance, and exploring the various options available.
IoT access technologies serve as the foundation for connecting devices to the internet. These technologies enable seamless communication between IoT devices and the cloud, facilitating data transfer and control. They can be categorized into wired and wireless access technologies.
Wired Access Technologies: Wired access technologies offer reliable and secure connectivity for IoT devices. Ethernet, for instance, has been a longstanding wired technology used in both industrial and residential settings. Power over Ethernet (PoE) further enhances the capabilities of Ethernet by providing power alongside data transmission. This section will explore the advantages and considerations of wired access technologies.
Wireless Access Technologies: Wireless access technologies have gained immense popularity due to their flexibility and scalability. Wi-Fi, Bluetooth, and Zigbee are among the most widely used wireless technologies in the IoT landscape. Each technology has its own strengths and limitations, making them suitable for different IoT use cases. This section will discuss the key features and applications of these wireless access technologies.
Cellular Connectivity for IoT: As IoT devices continue to expand, cellular connectivity has emerged as a critical access technology. Cellular networks provide wide coverage, making them ideal for remote and mobile IoT deployments. This section will explore cellular technologies such as 2G, 3G, 4G, and the emerging 5G, highlighting their capabilities and potential impact on IoT applications.
Security Considerations: With the proliferation of IoT devices, security becomes a paramount concern. This section will shed light on the security challenges associated with IoT access technologies and discuss measures to ensure secure and robust connectivity for IoT devices. Topics such as authentication, encryption, and device management will be explored.
IoT access technologies play a vital role in enabling seamless connectivity for IoT devices. Whether through wired or wireless options, these technologies bridge the gap between devices and the internet, facilitating efficient data exchange and control. As the IoT landscape continues to evolve, staying updated with the latest access technologies and implementing robust security measures will be key to harnessing the full potential of the Internet of Things.
Matt Conran
Highlights: Internet of Things Access Technologies
A practitioner in the tech industry should be familiar with the term Internet of Things (IoT). IoT continues to grow due to industries’ increasing reliance on the internet. Even though we sometimes don’t realize it, it is everywhere.
The Internet of Things plays an important role in the development of smart cities through its various implementations, such as real-time sensor data retrieval and automated task execution. The Internet of Things’ profound impact on our urban landscape, which is increasingly evident, means that these systems, equipped with numerous sensors, take action when specific thresholds are reached.
The Internet of Things, coined by computer scientist Kevin Ashton in 1999, is a network of connected physical objects that send and receive data. Smart fridges and mobile phones are examples of everyday objects, as are smart agriculture and smart cities, which span entire towns and industries.
IoT has revolutionized how we live and interact with our environment in just a few years. Households can benefit from IoT in the following ways:
- Convenience: Devices can be programmed and controlled remotely. Think about being able to adjust your home’s lighting or heating even when you’re miles away using your smartphone.
- Efficiency: Smart thermostats and lighting systems can optimize operations based on your usage patterns, saving energy and lowering utility bills.
- Security: In terms of security, IoT-enabled security systems and cameras can alert homeowners of potential breaches, and smart locks can recognize and grant access based on recognized users.
- Monitoring health metrics: Smart wearables can track health metrics and provide real-time feedback, potentially alerting users or medical professionals to concerning changes.
- Improved user experience: Devices can learn and adapt to users’ preferences, ensuring tailored and improved interactions over time.
IoT Access Technologies:
Wi-Fi:
One of the most widely used IoT access technologies is Wi-Fi. With high data transfer speeds and widespread availability, Wi-Fi enables devices to connect effortlessly to the internet. Wi-Fi allows for convenient and reliable connectivity, whether controlling your thermostat remotely or monitoring security cameras. However, its range limitations and power consumption can be challenging in specific IoT applications.
Cellular Networks:
Cellular networks, such as 3G, 4G, and now the emerging 5G technology, play a vital role in IoT connectivity. These networks offer broad coverage areas, making them ideal for IoT deployments in remote or rural areas. With the advent of 5G, IoT devices can now benefit from ultra-low latency and high bandwidth, paving the way for real-time applications like autonomous vehicles and remote robotic surgery.
Bluetooth:
Bluetooth technology has long been synonymous with wireless audio streaming, but it also plays a significant role in IoT connectivity. Bluetooth Low Energy (BLE) is designed for IoT devices, offering low power consumption and short-range communication. This makes it perfect for applications like wearable devices, healthcare monitoring, and intelligent home automation, where battery life and proximity are crucial.
Zigbee:
Zigbee is a low-power wireless communication standard designed for IoT devices. It operates on the IEEE 802.15.4 standard and offers low data rates and long battery life. Zigbee is commonly used for home automation systems, such as smart lighting, temperature control, and security systems. Its mesh networking capabilities allow devices to form a network and communicate with each other, extending the overall range and reliability.
LoRaWAN:
LoRaWAN (Long Range Wide Area Network) is a low-power, wide-area network technology for long-range communication. It enables IoT devices to transmit data over long distances, making it suitable for applications like smart agriculture, asset tracking, and environmental monitoring. LoRaWAN operates on unlicensed frequency bands, enabling cost-effective and scalable IoT deployments.
Example Product: Cisco IoT Operations Dashboard
### Streamlined Device Management
One of the standout features of the Cisco IoT Operations Dashboard is its capability to streamline device management. With a user-friendly interface, you can quickly onboard, monitor, and manage a wide array of IoT devices. The dashboard provides real-time visibility into device status, performance metrics, and potential issues, allowing for proactive maintenance and swift troubleshooting.
### Enhanced Security Measures
Security is a top priority when it comes to IoT devices, and the Cisco IoT Operations Dashboard excels in this area. It offers robust security protocols to protect your devices from vulnerabilities and cyber threats. Features such as encrypted communications, device authentication, and regular security updates ensure that your IoT ecosystem remains secure and resilient against attacks.
### Scalability for Growing IoT Networks
As your IoT network expands, managing an increasing number of devices can become challenging. The Cisco IoT Operations Dashboard is designed to scale effortlessly with your network. Whether you have a small deployment or a vast array of interconnected devices, the dashboard’s scalable architecture ensures that you can manage your IoT operations effectively, regardless of size.
### Integration and Interoperability
The Cisco IoT Operations Dashboard is built with integration and interoperability in mind. It seamlessly integrates with other Cisco products and third-party solutions, providing a cohesive and unified management experience. This interoperability allows for the aggregation of data from various sources, enabling comprehensive analytics and informed decision-making.
Related: Before you proceed, you may find the following post helpful:
Internet of Things Access Technologies
The Internet of Things consists of a network of several devices, including a range of digital and mechanical objects, with separate access to transfer information over a network. The word “thing” can also represent an individual with a heart- monitor implant or even a pet with an IoT-based collar.
The term “thing” reflects the association of “internet” to devices previously disconnected with internet access. For example, the alarm clock was never meant to be internet-enabled, but now you can connect it to the Internet. With IoT, the options are endless.
Examples: IoT Access Technologies
Then, we have IoT access technologies. The three major network access technologies for IoT Connectivity are Standard Wireless Access – WiFi, 2G, 3G, and standard LTE. We also have Private Long Range – LoRA-based platforms, Zigbee and SigFox. Mobile IoT Technologies – LTE-M, NB-IoT, and EC-GSM-IoT
The origins of the Internet, which started in the 1960s, look entirely different from our current map. It is now no longer a luxury but more of a necessity. It started with areas such as basic messaging, which grew to hold the elasticity and dynamic nature of the cloud, to a significant technological shift into the world of the Internet of Things (IoT): Internet of Things theory.
It’s not about buying and selling computers and connecting them anymore; it’s all about data, analytics, and new solutions, such as event stream processing.
Internet of Things Access Technologies: A New World
A World with the Right Connections
IoT represents a new world where previously unconnected devices have new communication paths and reachability points. This marks IoT as the next evolutionary phase of the Internet, building better customer solutions.
The revolutionary phase is not just a technical phase; ethical challenges now face organizations, society, and governments. In the past, computers relied on input from humans. We entered keystrokes, and the machine would perform an action based on the input.
The computer had no sensors that could automatically detect the world around it and perform a specific action based on that. IoT ties these functions together. The function could be behavioral, making the object carry out a particular task or provide other information. IoT brings a human element to technology, connecting physically to logic.
It’s all about data and analytics.
The Internet of Things is not just about connectivity. The power of IoT comes from how all these objects are connected and the analytics they provide. New analytics lead to new use cases that will lead to revolutionary ideas enhancing our lives. Sensors are not just put on machines and objects but also on living things. Have you heard of the connected cow? Maybe we should start calling this “cow computing” instead of “cloud computing.” For example, an agricultural firm called Chitale Group uses IoT technologies to keep tabs on herds to monitor their thirst.
These solutions will formulate a new type of culture, intertwining various connected objects and dramatically shifting how we interact with our surroundings. This new type of connectivity will undoubtedly formulate how we live and form the base of a new culture of connectivity and communication.
IoT Access Technologies: Data management – Edge, fog, and cloud computing
In the traditional world of I.T. networks, data management is straightforward. It is based on I.P. with a client/server model, and the data is in a central location. IoT brings new data management concepts such as Edge, Cloud, and Fog computing. However, the sheer scale of IoT data management presents many challenges. Low bandwidth of the last mile leads to high latency, and new IoT architectural concepts such as Fog computing, where you analyze data close to where it’s connected, are needed.
Like the cloud in Skype, Fog is on the ground and best suited for constrained networks that need contextual awareness and quick reaction. Edge computing is another term where the processing is carried out at the furthest point – the IoT device itself. Edge computing is often called Mist computing.
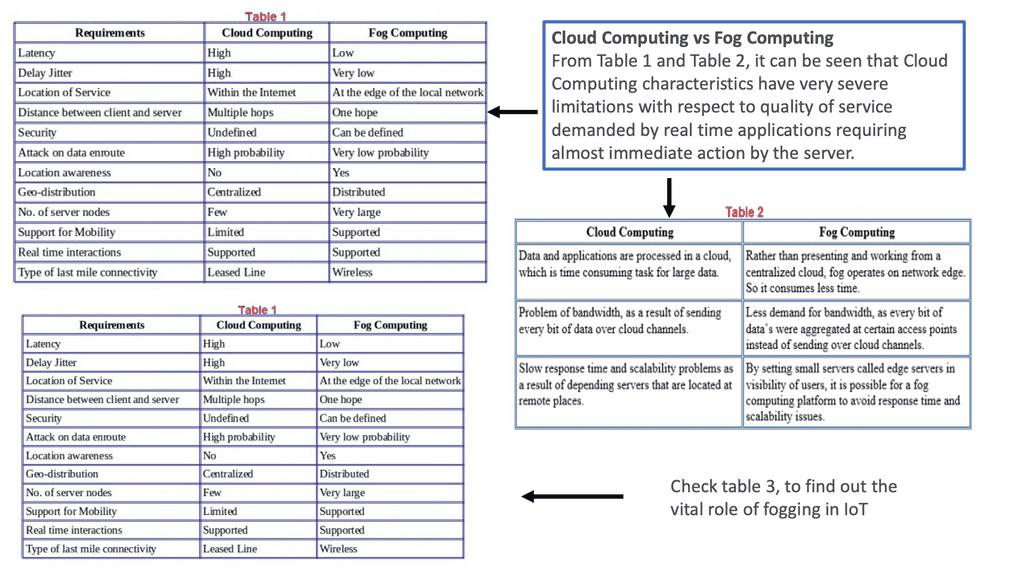
Cloud computing is not everything.
IoT brings highlights the concept that “cloud computing is not everything.” IoT will require onsite data processing; some data must be analyzed in real-time. This form of edge computing is essential when you need near-time results and when there isn’t time to send visual, speed, and location information to the cloud for instructions on what to do next. For example, if a dog runs out in front of your car, the application does not have the time for several round trips to the cloud.
Services like iCloud have had a rough few years. Businesses are worried about how secure their data will be when using one of the many cloud-based services available, especially after the iCloud data breach in 2014. However, with the rise of cloud security solutions, many businesses are starting to see the benefits of cloud technology, as they no longer have to worry about their data security.
The Internet is prone to latency, and we cannot fix it unless we shorten the links or change the speed of light. Connected cars require the capability to “think” and make decisions on the ground without additional hops to the cloud.
Fog Computing – Distributed Infrastructure
Fog computing is a distributed computing infrastructure between a network’s edge and the cloud. It is a distributed computing architecture designed to address the challenges of latency and bandwidth constraints introduced by traditional cloud computing. Fog computing decentralizes the computing process rather than relying on a single, centralized data center to store and process data. It enables data to be processed closer to the network’s edge.
Fog computing performs better than cloud computing in meeting the demands of emerging paradigms. However, batch processing is still preferred for high-end jobs in the business world, so it can only partially replace cloud computing. In conclusion, fog computing and cloud computing complement one another while having their advantages and disadvantages. Edge computing is crucial in the Internet of Things (IoT).
Security, confidentiality, and system reliability are research topics in the fog computing platform. Cloud computing will meet the needs of business communities with its lower cost based on a utility pricing model. In contrast, fog computing is expected to serve the emerging network paradigms that require faster processing with less delay and delay jitter. Fog computing will grow by supporting the emerging network paradigms that require faster processing with less delay and delay jitter.
Internet of Things Access Technologies: Architectural Standpoint
A ) From an architectural point of view, one must determine the technologies used to allow these “things” to communicate with each other. And the technologies chosen are determined by how the object is classified. I.T. network architecture has matured over the last decade, but IoT architectures bring new paradigms and a fresh approach. For example, traditional security designs consist of physical devices with well-designed modules.
B ) Although new technologies such as VM NIC Firewalls and other distributed firewalls other than IoT have dissolved the perimeter, IoT brings dispersed sensors outside the protected network, completely dissolving the perimeter to a new level.
C) When evaluating the type of network needed to connect IoT smart objects, one needs to address transmission range, frequency bands, power consumption, topology, and constrained devices and networks. The technologies used for these topologies include IEEE 802.15.4, IEEE 802.15.4g and IEEE 802.15.4e, IEEE 1901.2a, IEEE 802.11ah, LoRaWAN, and NB-IoT. The majority of them are wireless. Similar to I.T. networks, IoT networks follow Layer 1 (PHY), Layer 2 (MAC), Layer 3 (I.P.), etc. layers. And some of these layers require optimizations to support IoT intelligent objects.
IP/TCP/UDP in an IoT world
– The Internet Protocol (I.P.) is an integral part of IoT due to its versatility in dealing with the large array of changes in Layer 1 and Layer 2 to suit the last-mile IoT access technologies. I.P. is the Internet protocol that connects billions of networks with a well-understood knowledge base. Everyone understands I.P. and knows how to troubleshoot it.
– It has proven robust and scalable, providing a solid framework for bi-directional or unidirectional communication between IoT devices. Sometimes, the full I.P. stack may not be necessary as protocol overhead may exceed device data.
– More importantly, IPv6. Using I.P. for the last mile of constrained networks requires introducing a new mechanism and routing protocols, such as RLP and adaptation layers, to handle the constrained environments. In addition, routing protocol optimizations must occur for constrained devices and networks. This is where we see the introduction of the IPv6 RPL protocol. IPv6 RPL protocol is a distance-vector routing protocol specifically designed for IoT intelligent objects.
– Optimizations are needed at various levels, and control plane traffic must be kept to a minimum, leading to new algorithms such as On-Demand Distance Vector. Standard routing algorithms learn the paths and store the information for future use. This works compared to AODV, which does not send a message until a route is needed.
Both TCP and UDP have their place in IoT:
TCP and UDP will play a significant role in the IoT transport layer. TCP for guaranteed delivery or UDP to leave the handling to a higher layer. The additional activities TCP brings to make it a reliable transport protocol come at the overhead cost per packet and session.
On the other hand, UDP is connectionless and often used for performance, which is more critical than packet retransmission. Therefore, a low-power and Lossy network (LLN) may be better suited than UDP and a more robust cellular network for TCP.
Session overhead may not be a problem on everyday I.T. infrastructures. Still, it can cause stress on IoT-constrained networks and devices, especially when a device needs only to send a few bytes per bytes of data per transaction. IoT Class 0 devices that only send a few bytes do not need to implement a full network protocol stack. For example, small payloads can be transported on top of the MAC layer without TCP or UDP.
**Ethical Challenges**
What are the ethical ramifications of IoT? In the Cold War, everyone was freaked out about nuclear war; now, it’s all about data science. We are creating billions of connected “things,” and we don’t know what’s to come. The responsibilities around the ethical framework for IoT and the data it generates fall broadly on individual governments and society.
These are not technical choices; they are socially driven. This might scare people and hold them back with IoT, but if you look at technology, it’s been a fantastic force for good. One should not resist IoT and the use cases it will offer our lives. However, I don’t think it will work out well for you if you do.
New technologies will always have risks and challenges. But if you reflect on and look at original technologies, such as the wheel, they created new jobs rather than destroyed old ones. IoT is the same. It’s about being on the right side of history and accepting it.
Example Product: Cisco Edge Device Manager
### What is Cisco Edge Device Manager?
Cisco Edge Device Manager is a cutting-edge platform designed to simplify the management of edge devices. It provides a unified interface for monitoring, configuring, and troubleshooting devices located at the edge of the network. By leveraging Cisco EDM, businesses can ensure optimal performance, reduce downtime, and enhance security across their edge infrastructure.
### Key Features and Benefits
#### Seamless Integration
One of the standout features of Cisco Edge Device Manager is its seamless integration with the Cisco IoT Operations Dashboard. This synergy allows for a centralized view of all IoT devices, enabling efficient management and streamlined operations.
#### Enhanced Security
Cisco EDM prioritizes security, offering advanced features such as automated firmware updates, real-time threat detection, and secure communication channels. These measures ensure that your edge devices are protected against potential cyber threats.
#### Scalability
Whether you have a handful of devices or thousands, Cisco Edge Device Manager scales effortlessly to meet your needs. Its flexible architecture supports the growth of your IoT infrastructure without compromising performance or reliability.
### Practical Applications
Cisco Edge Device Manager is not just a tool; it’s a game-changer for various industries. Here are a few practical applications:
– **Manufacturing**: Monitor and manage factory equipment in real-time to enhance productivity and reduce maintenance costs.
– **Retail**: Ensure seamless operation of point-of-sale systems and inventory tracking devices, improving customer experience.
– **Healthcare**: Maintain the performance and security of medical devices, ensuring patient safety and data integrity.
### Getting Started with Cisco Edge Device Manager
Setting up Cisco Edge Device Manager is straightforward. Begin by integrating it with your existing Cisco IoT Operations Dashboard. Next, configure your edge devices through the intuitive interface, and start monitoring their performance and security. Cisco provides detailed documentation and support to guide you through each step.
Cisco and IoT
One technology that has significantly advanced IoT is Cisco’s LoRaWAN. LoRaWAN, short for Long Range Wide Area Network, is a low-power, wide-area network protocol for long-range communication between IoT devices.
It operates in the sub-gigahertz frequency bands, providing an extended communication range while consuming minimal power. This makes it ideal for applications that require long-distance connectivity, such as smart cities, agriculture, asset tracking, and industrial automation.
Cisco’s Contribution: Cisco, a global leader in networking solutions, has embraced LoRaWAN technology and has been at the forefront of driving its adoption. The company has developed a comprehensive suite of LoRaWAN solutions, including gateways, sensors, and network infrastructure, enabling businesses to leverage the power of IoT.
Example Use Case: Cisco’s LoRaWAN-compliant solution.
With Cisco’s LoRaWAN-compliant solution, IoT sensors and endpoints can be connected cheaply across cities and rural areas. Low power consumption also extends battery life up to several years. The solution operates in the 800–900 MHz ISM band around the globe as part of Cisco’s LoRaWAN (long-range wide-area network) solution.
The Cisco Industrial Asset Vision solution includes it and a stand-alone component. Monitoring equipment, people, and facilities with LoRaWAN sensors improve business resilience, safety, and efficiency.
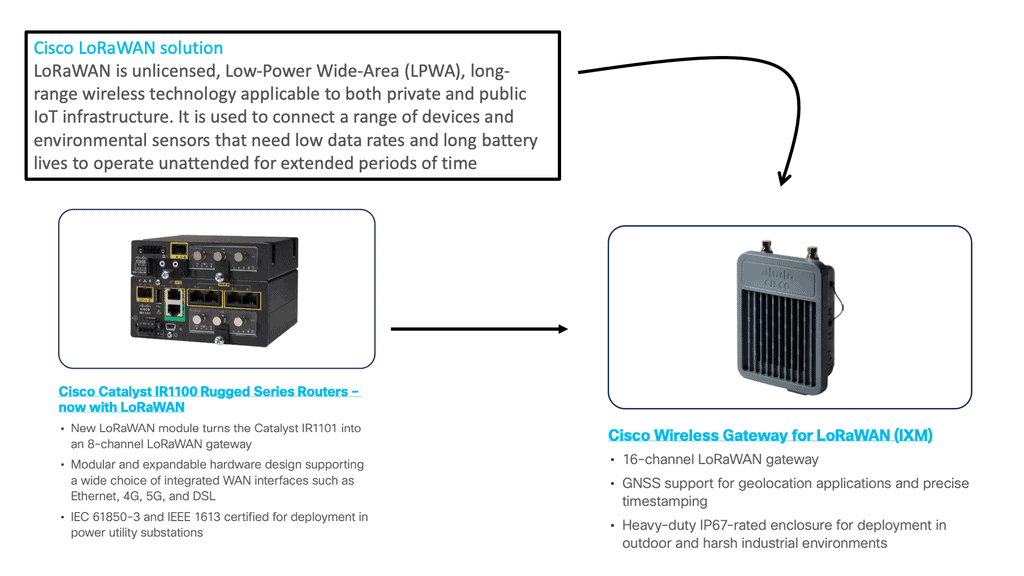
Benefits of Cisco’s LoRaWAN:
1. Extended Range: With its long-range capabilities, Cisco’s LoRaWAN enables devices to communicate over several kilometers, surpassing the limitations of traditional wireless networks.
2. Low Power Consumption: LoRaWAN devices consume minimal power, allowing them to operate on batteries for an extended period. This makes them ideal for applications where the power supply is limited or impractical to install.
3. Scalability: Cisco’s LoRaWAN solutions are highly scalable, accommodating thousands of devices and ensuring seamless communication between them. This scalability makes it suitable for large-scale deployments, such as smart cities or industrial IoT applications.
4. Secure Connectivity: Security is a top priority in any IoT deployment. Cisco’s LoRaWAN solutions incorporate robust security measures, ensuring data integrity and protection against unauthorized access.
Cisco’s LoRaWAN Use Cases:
1. Smart Agriculture: LoRaWAN allows farmers to monitor soil moisture, temperature, and humidity, optimize irrigation, and reduce water consumption. Cisco’s LoRaWAN solutions provide reliable connectivity to enable efficient farming practices.
2. Asset Tracking: From logistics to supply chain management, tracking assets in real-time is crucial. Cisco’s LoRaWAN solutions enable accurate and cost-effective asset tracking, enhancing operational efficiency.
3. Smart Cities: LoRaWAN is vital in building smart cities. It allows municipalities to monitor and manage various aspects, such as parking, waste management, and street lighting. Cisco’s LoRaWAN solutions provide the necessary infrastructure to support these initiatives.
As the IoT ecosystem expands, the choice of access technologies becomes critical to ensure seamless connectivity and efficient data exchange. Wi-Fi, cellular networks, Bluetooth, Zigbee, and LoRaWAN are examples of today’s diverse IoT access technologies.
By understanding the strengths and limitations of each technology, businesses and individuals can make informed decisions about which access technology best suits their IoT applications. As we embrace the connected future, IoT access technologies will continue to evolve, enabling us to unlock the full potential of the Internet of Things.
IoT Access Technologies Closing Points
Wi-Fi is one of the most widely used access technologies in IoT. It offers high data rates, making it ideal for applications that require significant bandwidth, such as video streaming from security cameras or data transfers from smart appliances. However, Wi-Fi networks typically have limited range, which may necessitate additional infrastructure for widespread IoT deployments. Its widespread adoption in residential and commercial settings makes Wi-Fi a popular choice for many IoT applications
Cellular networks provide a robust solution for IoT devices that require mobility and extensive coverage. Technologies like 4G LTE and the emerging 5G networks offer high-speed data connections over large distances, making them perfect for applications such as connected vehicles and remote monitoring systems. The ability to maintain connectivity while moving and the potential for low-latency communication with 5G make cellular networks a pivotal component in the IoT landscape.
Low Power Wide Area Networks (LPWAN) are designed specifically for IoT applications that require long-range connectivity with minimal power consumption. Technologies such as LoRaWAN and Sigfox excel in scenarios where devices need to operate for extended periods without frequent battery replacements, such as in environmental monitoring or smart agriculture. These networks typically offer lower data rates, but their energy efficiency and extensive coverage make them ideal for specific IoT use cases.
For IoT applications that require short-range communication, Bluetooth and Zigbee are popular choices. Bluetooth is widely used in personal devices like wearables and health monitors due to its low power consumption and ease of integration. Zigbee, on the other hand, is often employed in smart home ecosystems, providing reliable mesh networking capabilities that enhance device-to-device communication within a limited area. Both technologies offer solutions for specific IoT scenarios where proximity and power efficiency are priorities.
Summary: Internet of Things Access Technologies
The Internet of Things (IoT) has become pervasive in our ever-connected world, transforming how we live, work, and interact with technology. As the IoT continues to expand, it is crucial to understand the access technologies that enable its seamless integration. This blog post delved into IoT access technologies, highlighting their importance, benefits, and potential challenges.
What are IoT Access Technologies?
IoT access technologies encompass the various means through which devices connect to the internet and communicate with each other. These technologies provide the foundation for IoT ecosystems, enabling devices to exchange data and perform complex tasks. From traditional Wi-Fi and cellular networks to emerging technologies like LPWAN (Low Power Wide Area Network) and 5G, the landscape of IoT access technologies is diverse and constantly evolving.
Traditional Access Technologies
Wi-Fi and cellular networks have long been the go-to options for connecting IoT devices. Wi-Fi offers high bandwidth and reliable connectivity within a limited range, making it suitable for home and office environments. On the other hand, cellular networks provide wider coverage but may require a subscription and can be costlier. Both technologies have strengths and limitations, depending on the specific use case and requirements.
The Rise of LPWAN
LPWAN technologies have emerged as a game-changer in IoT connectivity. These low-power, wide-area networks offer long-range coverage, low energy consumption, and cost-effective solutions. LPWAN technologies like LoRaWAN and NB-IoT are ideal for applications that require battery-powered devices and long-range connectivity, such as smart cities, agriculture, and asset tracking.
The Promise of 5G
The advent of 5G technology is set to revolutionize IoT access. With its ultra-low latency, high bandwidth, and massive device connectivity, 5G opens up a world of possibilities for IoT applications. Supporting many devices in real-time with near-instantaneous response times unlocks new use cases like autonomous vehicles, remote healthcare, and smart industries. However, the deployment of 5G networks and the associated infrastructure pose challenges that must be addressed for widespread adoption.
Conclusion:
Internet of Things access technologies form the backbone of our interconnected world. From traditional options like Wi-Fi and cellular networks to emerging technologies like LPWAN and 5G, each has unique features and suitability for IoT applications. As the IoT expands, it is essential to leverage these technologies effectively, ensuring seamless connectivity and bridging the digital divide. By understanding and embracing IoT access technologies, we can unlock the full potential of the Internet of Things, creating a smarter and more connected future.